Soft AE Symposium 2025
Soft Materials, Autonomous Experimentation & Science Policy
2025 Symposium
Friday, May 2, 2025
9:00 am - 4:30 pm
Glandt Forum
Singh Center for Nanotechnology
3205 Walnut Street, Philadelphia PA
Funded by NSF Award #2152205
9:00 am
Chinedum Osuji, PhD
NRT Soft AE Director and PI
9:10 am
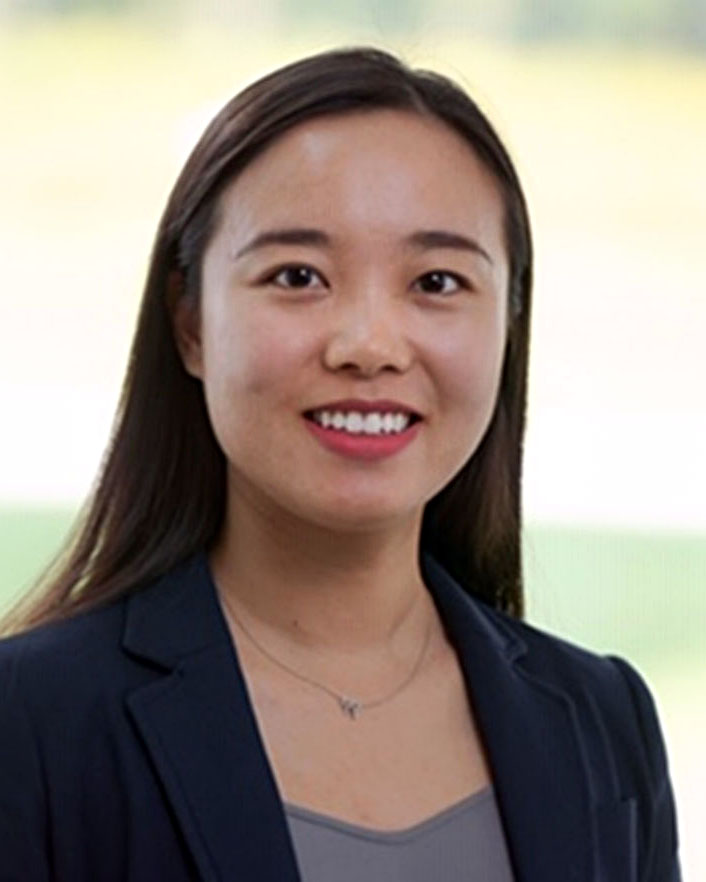
Engineering Electronic Polymers using Self-Driving Laboratory
Jie Xu, PhD, Argonne National Lab
The development of electronic polymers has lagged behind the rapidly growing demand for advanced materials in flexible devices, large-scale printable electronics, and sustainable energy applications. This slow progress stems from the vast design space and complex processing conditions required, making precise design a formidable challenge. Balancing critical properties—like electronic mobility, strength, ionic conductivity, sustainability, and processability—further complicates the development pipeline. To address these challenges, we are pioneering new approaches to accelerate the electronic polymer development pipeline. While AI-driven materials research has seen rapid advances, applying these technologies to electronic polymer design remains challenging, particularly due to the limited data availability stemming from the lengthy design-make-test-analyze cycle in electronics. Our work focuses on accelerating the design of functional polymers by leveraging artificial intelligence (AI) and automated robotic experimentation. This talk will highlight research conducted in our self-driving lab, Polybot, covering topics from the inverse discovery of electrochromic polymer structures, the controlled assembly of conducting polymers through solution processing, and the discovery of design principles for mixed-conducting polymers in electrochemical transistors. We will also discuss ongoing efforts to evolve Polybot into a more adaptive system with enhanced human-machine interfaces and as a community resource by building a specialized electronic polymer database.
Jie Xu is a scientist at Argonne National Lab. Her research focuses on precision engineering of functional polymers through molecular packing structure engineering, chemical design, and self-driving laboratories (https://www.anl.gov/cnm/polybot). Jie earned her PhD in chemistry from Nanjing University, specializing in nanoconfined soft matter, and completed postdoctoral training at Stanford in stretchable electronics. She received the Materials Research Society Postdoctoral Award and is named to the MIT Technology Review’s list of Innovators Under 35, Newsweek list of America’s Greatest Disruptors as a budding disruptor, and 2023 Polymeric Materials: Science and Engineering Early Investigator Honoree by the American Chemical Society.
10:30 am
Coffee break
10:50 am
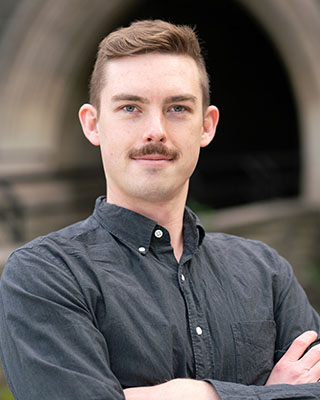
Establishing the Data-Driven Organic Materials Lab
Martin Seifrid, PhD, North Carolina State University
To address pressing challenges in energy and healthcare, we must accelerate the pace of scientific discovery through digital technology, data-driven science, and automation. In this talk, I will discuss my group’s efforts to combine experimental and computational tools to accelerate the design and discovery of organic materials. I will provide an overview of how to build a self-driving lab, from both digital and experimental perspectives. Finally, I will provide a glimpse into my lab’s current research directions.
Prof. Martin Seifrid is an assistant professor in the Department of Materials Science and Engineering at North Carolina State University. His group develops self-driving laboratories – automated experiments guided by machine learning – and leverages them to design precisely controlled organic mixed ionic-electronic conducting materials with applications in sensing, energy storage, healthcare, and neuromorphic computing.
Prof. Seifrid received his Ph.D. in Chemistry from the University of California, Santa Barbara in 2019. He worked in the Center for Polymers and Organic Solids from 2014 to 2019 under the supervision of Professor Guillermo C. Bazan. In October 2019, he joined the group of Prof. Alán Aspuru-Guzik at the University of Toronto for a postdoctoral fellowship. There, he worked on self-driving laboratories, autonomous molecular design, automated synthesis and characterization, and organic lasing materials.
11:35 am
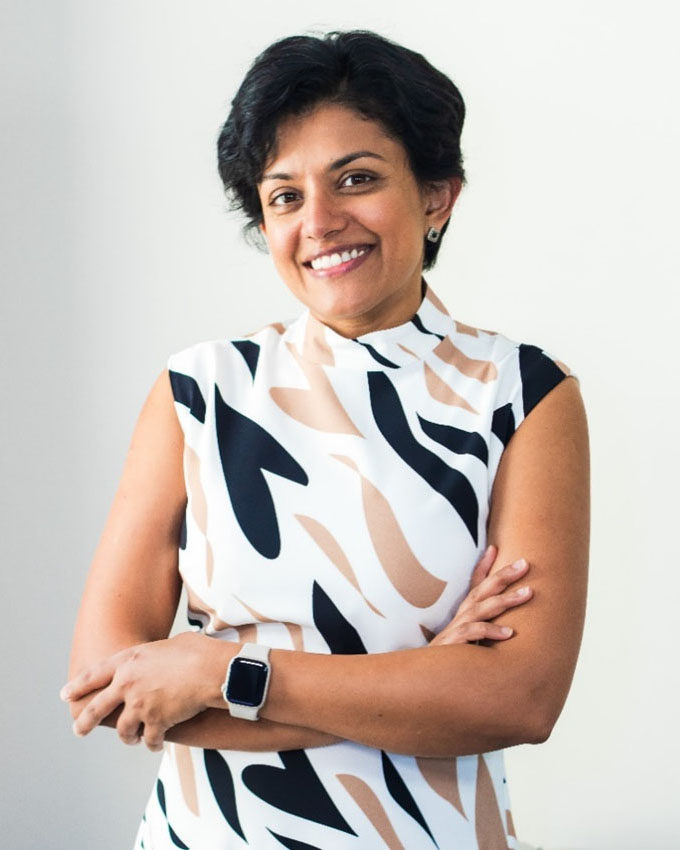
Convergence of Molecular Modeling, Simulations, Machine Learning, and Soft Materials Science in Research and Interdisciplinary Education
Arthi Jayaraman, PhD, University of Delaware
Arthi Jayaraman is currently a full professor in the Departments of Chemical and Biomolecular Engineering and Materials Science and Engineering at the University of Delaware (UD), Newark. She is also the director for an NSF-funded NRT graduate traineeship program on ‘Computing and Data Science Training for Materials Innovation, Discovery, and Analytics’. She currently serves as associate editor for Macromolecules and also served as the inaugural deputy editor for ACS Polymers Au from 01/2021 – 12/2023. Jayaraman’s research expertise is in the development and application of computational techniques to study polymer nanocomposites, blends, solutions, and biomaterials. She has received the following honors: ACS PMSE Fellowship (2025), UD College of Engineering Faculty Award for Excellence in Teaching (2023), AIChE COMSEF Impact Award (2021), APS Fellowship (2020), ACS PMSE Young Investigator (2014), AIChE COMSEF Young Investigator Award (2013), University of Colorado (CU) Provost Faculty Achievement Award (2013), Department of Energy (DOE) Early Career Research Award (2010), and CU Department of Chemical and Biological Engineering’s outstanding undergraduate teaching award (2011) and graduate teaching award (2014).
12:20 pm
Group Photo
12:30 pm
Lunch
1:30 pm
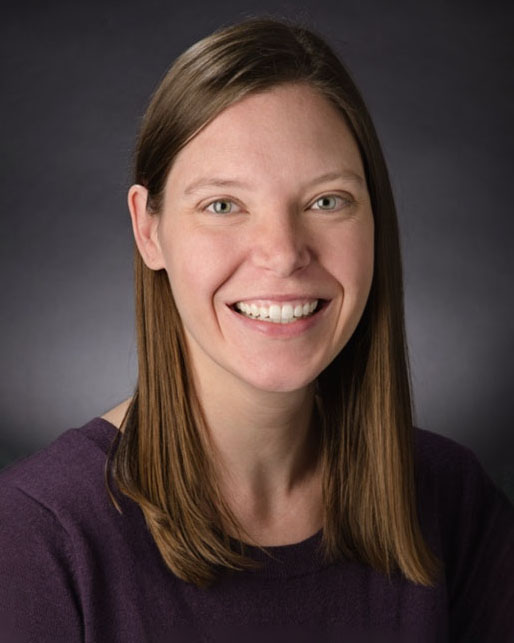
Improving Machine Learning with Polymer Science
Debra J. Audus, PhD, NIST
Machine learning as applied to polymer science has shown immense progress, mostly in areas where there are existing large datasets or where datasets can be generated quickly. However, there are numerous interesting problems where the dataset sizes are too small or the need to understand the science behind the machine learning prediction is essential. Here, we aim to tackle both problems by incorporating domain knowledge, in the form of polymer theories, into machine learning models. First, we consider a toy system of polymers in different solvent qualities and compare several methods for incorporating theory into machine learning using a simple, imperfect but easily interpretable theory. Second, we consider the phase behavior of polystyrene in cyclohexane using the foundational Flory-Huggins theory for guidance. We compare three theory informed machine learning methods along with a theory constrained machine learning method. Relative trade-offs and implications on explainability are explored.
Dr. Debra J. Audus is the project leader of the Polymer Analytics Project in the Materials Science and Engineering Division at NIST. She came to NIST in 2013 after receiving her PhD in Chemical Engineering at University of California, Santa Barbara and BS from Cornell University. Her research focuses on improving machine learning by embedding polymer science, explainable machine learning, polymer databases and using both theory and simulation to understand polymer physics. She is an active member of the American Physical Society, American Chemical Society and Materials Research Data Alliance, for which she organized the annual meeting in 2024. In 2023, she received the Communications Materials Outstanding Referee award.
2:50 pm
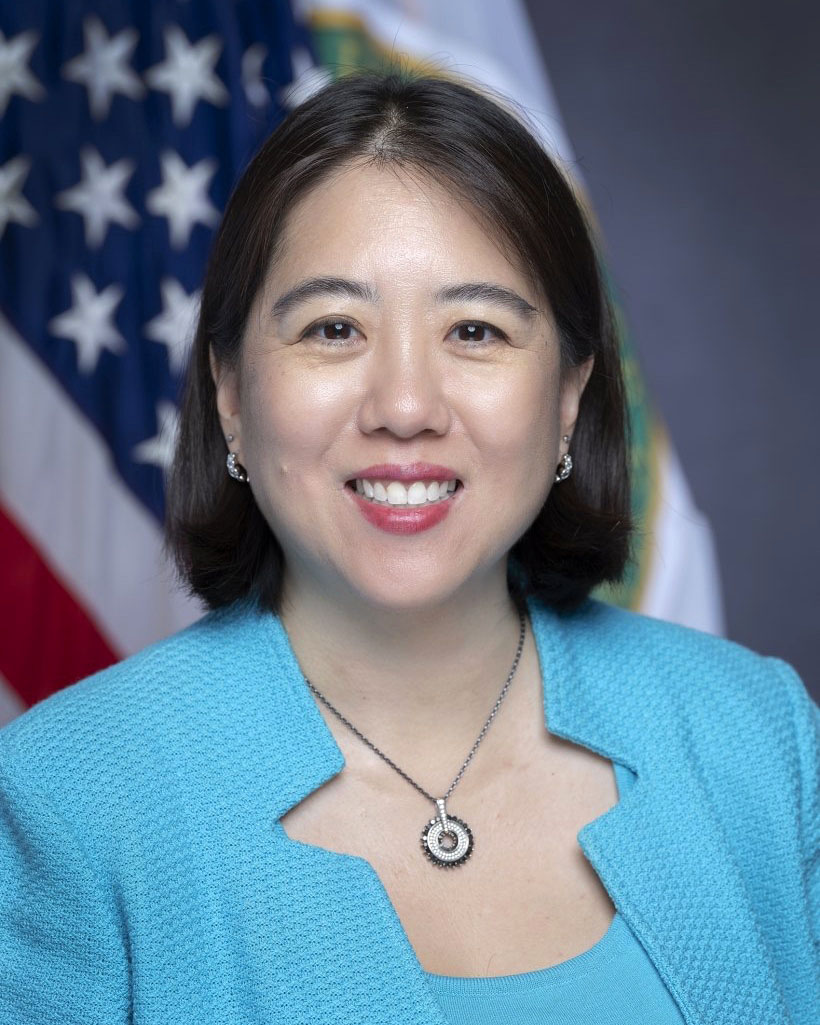
The Key to Technology Commercialization is not in Technology Readiness
Vanessa Chan, PhD, University of Pennsylvania
In our academic trainings we are focused on tackling the hard science and technology issues. We are measured by the number of peer-reviewed journal articles that we publish, and these are based on the rigor of our scientific research. Traditionally the likelihood of a technology making it to market has been measured by its TRLs (Technology Readiness Levels) which assesses how mature the technology is. The problem is that TRLs do not consider non-technology barriers that must be overcome for a technology to be used in the real world. Barriers such as unit cost economics, regulatory hurdles, availability of supply chain etc. A complementary framework, ARLs (Adoption Readiness Levels) was created to capture the non-technical challenges that technologies must overcome for full-scale deployment into the marketplace. By assessing TRLs in parallel with ARLs this will help steward technologies along the research, development, demonstration & deployment (RDD&D) or commercialization continuum.
Vanessa Chan is an accomplished entrepreneur, angel investor, speaker and educator renowned for her expertise in commercializing technologies. She is the inaugural Vice Dean of Innovation & Entrepreneurship at the University of Pennsylvania's Engineering School and the Jonathan & Linda Brassington Professor of Practice. In 2021, Dr. Chan was appointed by President Biden to serve as the Chief Commercialization Officer at the U.S. Department of Energy and the Director of the Office of Technology Transitions. In this role, she was instrumental in nationally advancing clean energy technologies, notably through the "Pathways to Commercial Liftoff" reports, which provided road maps scaling critical technologies like nuclear and geothermal. She also led the creation of a new framework and language for commercialization, "Adoption Readiness Levels" which is being used throughout federal government, incubators, VCs and the private sector to improve commercialization outcomes. Her impact was recognized through her inclusion as one of the world's 100 most influential business leaders in climate in TimelO0's 2024 list.
Before her tenure at the Department of Energy, Vanessa was a partner at McKinsey & Company where she co-led the innovation practice, helping large publicly traded companies commercialize their technologies. Following her time at McKinsey, she founded re.design, a company dedicated to reinventing consumer products to solve everyday challenges selling her products on QVC. Dr. Chan earned her Ph.D. from MIT and a B.S.E. from the University of Pennsylvania, both in Materials Science and Engineering. She is a working parent and in her personal time, Dr. Chan is the co-owner of a pottery studio, an avid mountain biker, yoga practitioner, skier, and world traveler.
3:35 pm
Russell Composto, PhD
NRT Soft AE Associate Director and co-PI
3:40 pm
Reception